31. March 2025
Unlocking Smarter Tech: How Less Data Can Fuel More Accurate Insights In Ai Systems
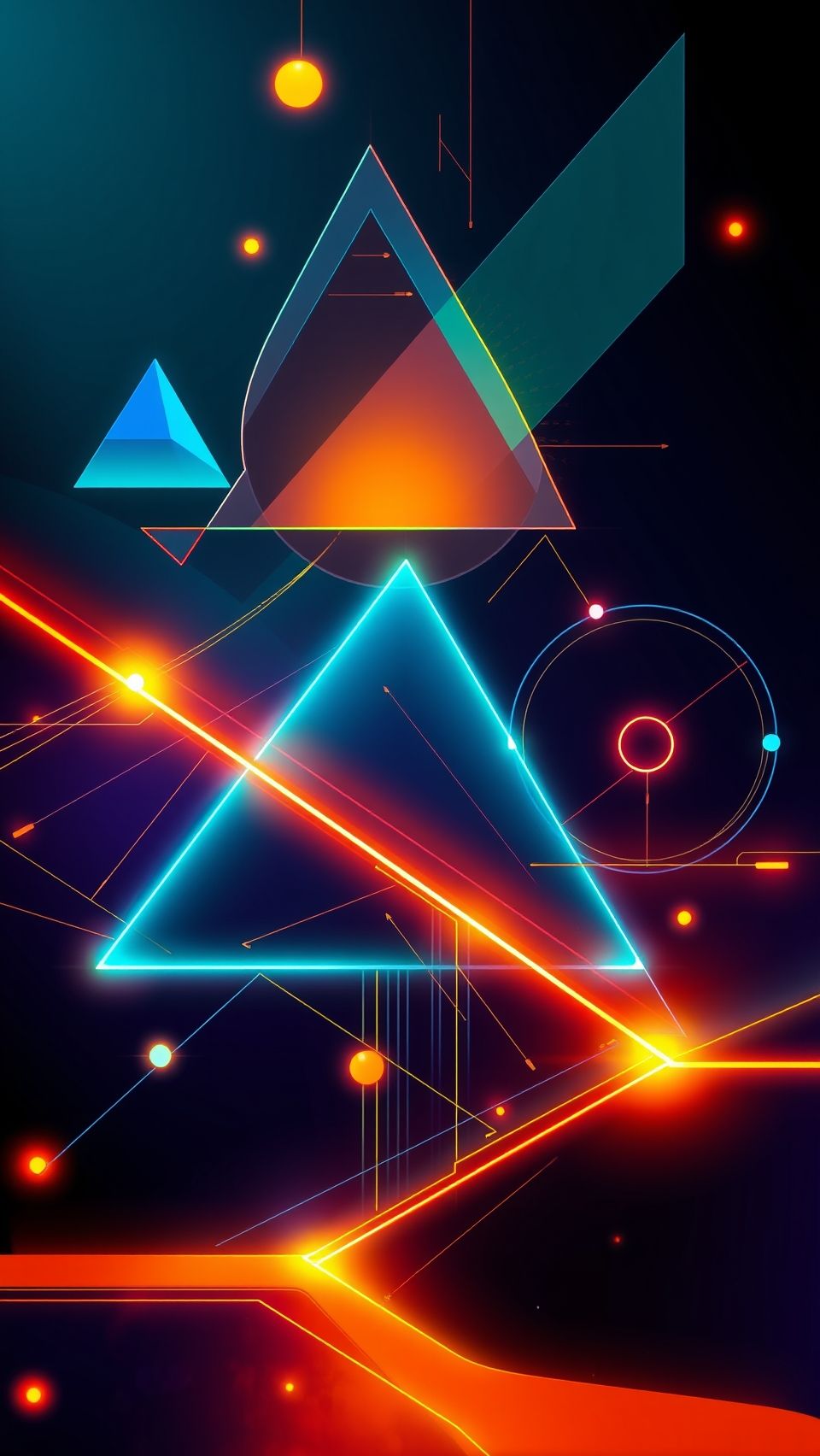
The Art of Information Retrieval in AI Systems: Why Less Can Be More
Artificial intelligence (AI) has made tremendous progress in recent years, transforming the way we interact with technology. One area where AI excels is in natural language processing (NLP), which enables machines to understand and generate human-like text. Language models are a crucial component of NLP, allowing AI systems to answer questions, summarize documents, and even create original content.
Retrieval-Augmented Generation (RAG) is an approach to building AI systems that combines a language model with an external knowledge source. In essence, the AI first searches for relevant documents related to a user’s query, and then uses the language model to generate a response based on those retrieved documents. This approach has proven effective in various applications, including question answering, text summarization, and content generation.
However, recent research has revealed that the quality of information retrieval can have a significant impact on the accuracy and efficiency of AI systems. In this article, we will explore the findings of a study that suggests that focusing on fewer, higher-quality documents can lead to better results than relying on a large volume of mixed-quality information.
A recent study published in a leading NLP conference explored the impact of document quality on RAG systems. The researchers examined how different types of retrieved documents affected the accuracy and efficiency of AI-generated answers. They found that while simply increasing the number of retrieved documents improved answer accuracy, it also led to decreased efficiency and increased computational overhead.
The study’s findings revealed that when all documents were considered equally relevant, the model’s performance suffered from reduced accuracy and increased errors. However, when only the top-ranked documents were considered, the model’s performance improved significantly, with a notable increase in accuracy and a decrease in computational overhead.
This research has important implications for the future of AI systems that rely on external knowledge. It suggests that designers of RAG systems should prioritize smart filtering and ranking of documents over sheer volume. Instead of fetching 100 possible passages and hoping the answer is buried in there somewhere, it may be wiser to fetch only the top few highly relevant ones.
Focusing on fewer documents leads to better results due to several reasons. Firstly, it reduces computational overhead, allowing the system to process more requests in less time. Secondly, it improves accuracy by minimizing the introduction of conflicting information or noise into responses. Finally, it increases efficiency by enabling the model to focus more easily on key points and nuances of input questions.
The findings from this study have significant implications for future research in AI systems that rely on external knowledge. It highlights the need for smarter filtering and ranking mechanisms to ensure that only the most relevant documents are considered. Some potential directions for future research include developing better retriever systems, improving language models, and developing techniques for handling conflicting information.
Developing better retriever systems could lead to more effective document identification and retrieval. Improving language models would enable them to process retrieved documents more robustly and accurately. Handling conflicting information is essential for ensuring that AI-generated answers are accurate and reliable.
The era of giant context windows, where AI systems can process vast amounts of text simultaneously, emphasizes the need to focus on the quality of the information rather than just its quantity. By prioritizing smart filtering and ranking mechanisms over sheer volume, we can create AI systems that are not only accurate but also efficient and reliable.
As researchers and developers, we must continue to push the boundaries of what is possible with AI systems. By embracing the findings from this study, we can build more effective RAG systems that rely on fewer, higher-quality documents to generate accurate and reliable answers.