24. January 2025
Revolutionary Ai Model Outperforms Traditional Rivals With Groundbreaking Efficiency
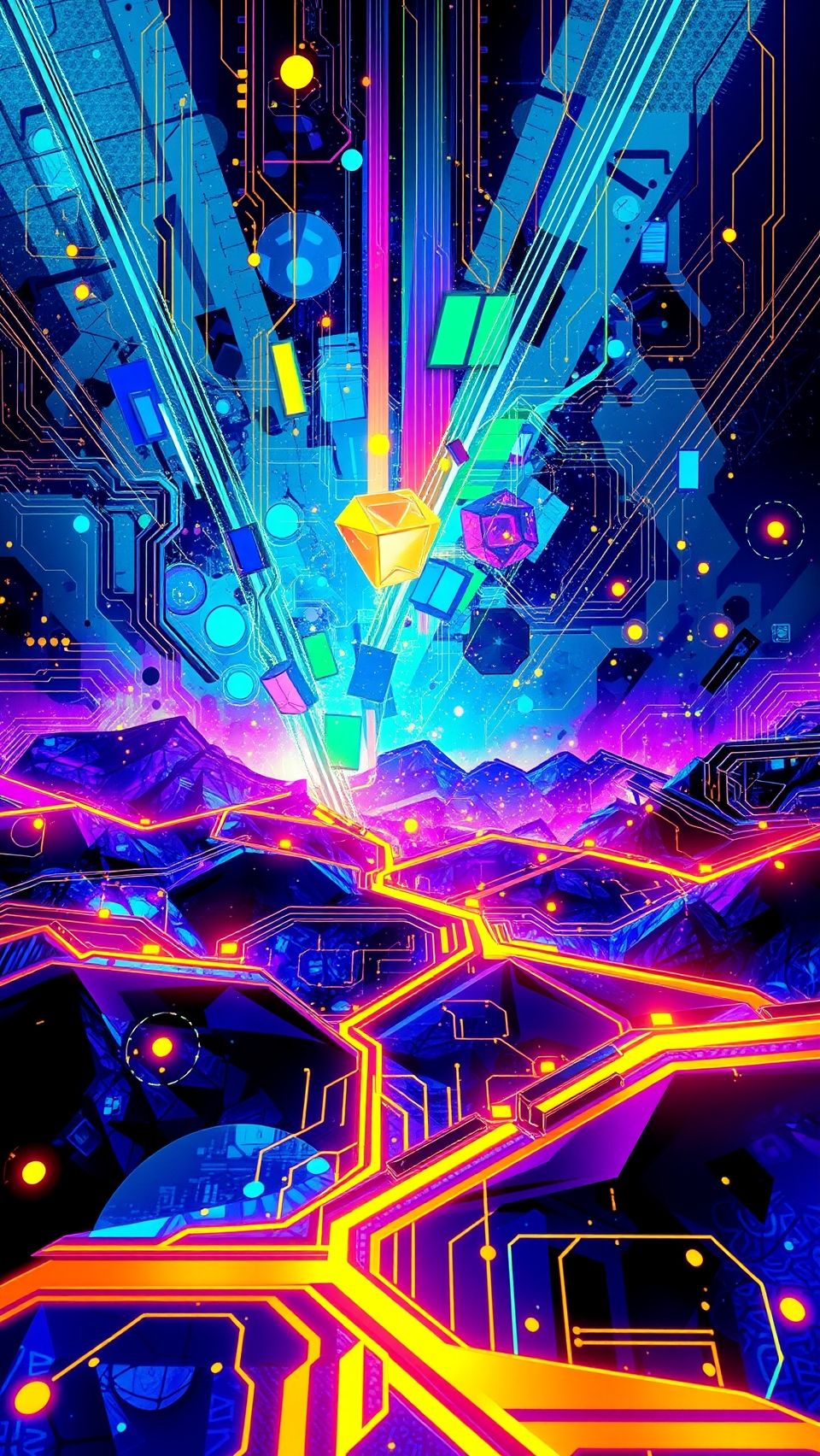
DeepSeek-V3, a new generative AI model, has overcome several challenges in traditional models. By employing a Mixture-of-Experts (MoE) architecture, it selectively activates 37 billion parameters per token, ensuring strategic resource allocation and achieving high performance without the hardware demands of traditional models.
In contrast to traditional LLMs that rely on Transformer architectures and memory-intensive caches, DeepSeek-V3 employs an innovative Multi-Head Latent Attention (MHLA) mechanism. MHLA transforms how KV caches are managed by compressing them into a dynamic latent space using “latent slots.” These slots serve as compact memory units, distilling only the most critical information while discarding unnecessary details.
DeepSeek-V3 uses mixed precision training with FP8, which reduces GPU memory usage and speeds up training without compromising numerical stability and performance. The model also employs DualPipe to overlap computation and communication between GPUs, reducing idle periods and improving the computation-to-communication ratio.
The model was trained on an extensive dataset of 14.8 trillion high-quality tokens over approximately 2.788 million GPU hours on Nvidia H800 GPUs, with a total cost of around $5.57 million. This stark contrast underscores DeepSeek-V3’s efficiency, achieving cutting-edge performance with significantly reduced computational resources and financial investment.
DeepSeek-V3’s innovations deliver superior reasoning capabilities, outperforming industry leaders in multi-step problem-solving and contextual understanding. The model achieves high performance without sacrificing efficiency or resources, making it more cost-effective and sustainable. This approach makes it a practical solution for organizations and developers that combines affordability with cutting-edge capabilities.