10. January 2025
Google Deepmind Develops Game-Changing Benchmark To Tackle Language Model Factuality Issues
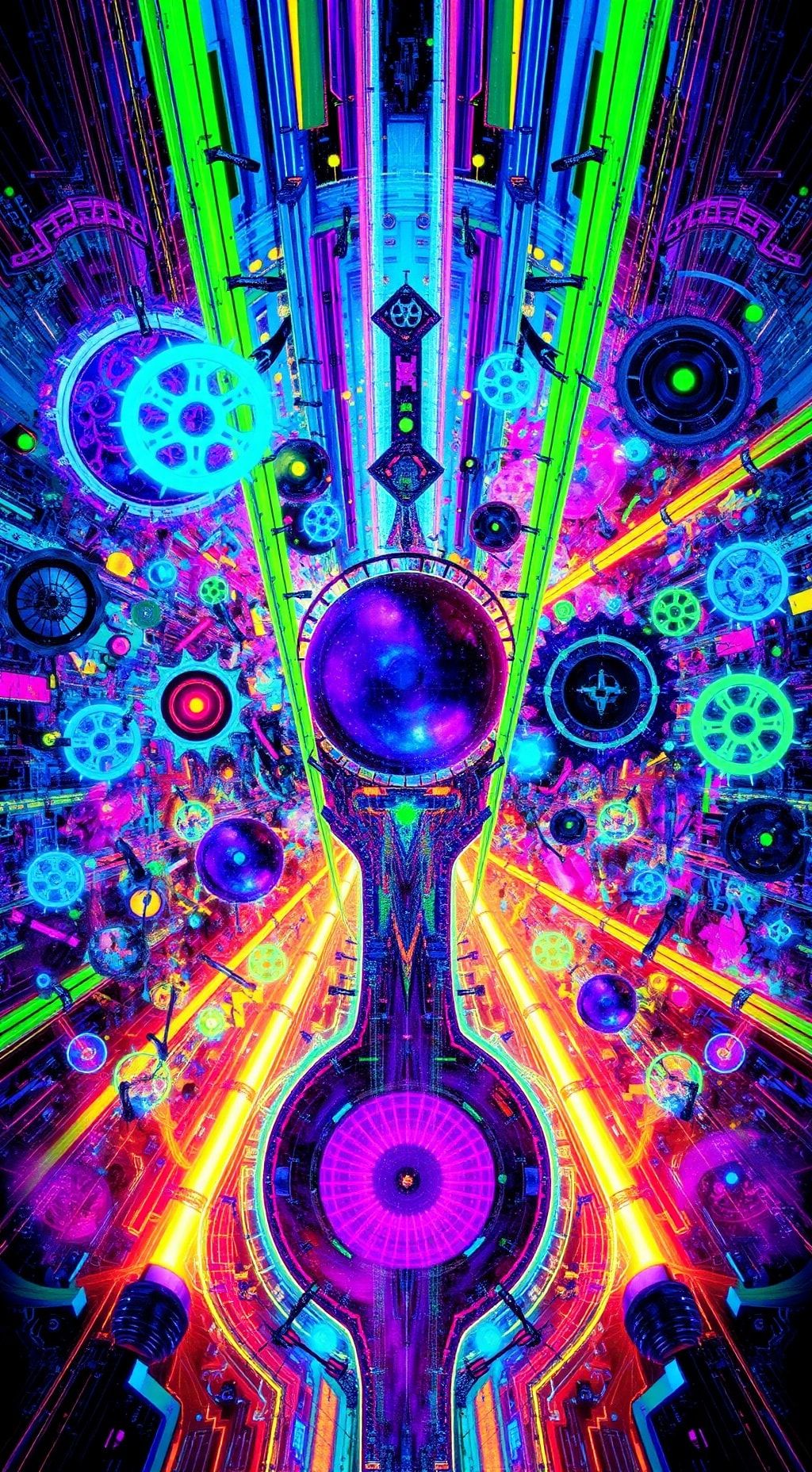
Google DeepMind researchers have made a significant breakthrough in improving the factuality of large language models (LLMs), which continue to struggle with providing accurate responses. A new benchmark called FACTS Grounding evaluates LLMs’ ability to generate factually accurate responses based on long-form documents.
The benchmark assesses not only the accuracy of responses but also their detail and usefulness, judging whether answers provide relevant information that is directly supported by the document. This approach aims to weed out hallucinations, or factually inaccurate responses, which have plagued LLMs in the past.
To develop the FACTS Grounding benchmark, researchers created a dataset of 1,719 examples, each requiring long-form responses based on context provided in documents. The dataset includes a system prompt, task, and long document, which must be processed to create a subsequent long-form response that is comprehensive and directly attributable to the document.
The benchmark’s release has sparked excitement among researchers, with Gemini 2.0 Flash topping the leaderboard with a factuality score of 83.6%. Other top-performing models include Google’s Gemini 1.0 Flash and OpenAI’s GPT-4o, which ranked above 61.7% in terms of accuracy.
Researchers believe that FACTS Grounding fills a gap in evaluating LLM behaviors pertaining to factuality, particularly in comparison to benchmarks that focus on narrower use cases such as summarization alone. By incorporating diverse documents and user requests, the benchmark allows for a more comprehensive evaluation of model performance.
Three different LLM judges were used to evaluate each example, resulting in an average score that took into account any biases towards other models in the same family. This approach helped ensure that the responses were indeed factual and not hallucinatory.
The introduction of FACTS Grounding is a significant step forward in improving the factuality of LLMs. By developing comprehensive benchmarking methods and continuous research, Google DeepMind aims to improve AI systems and make them more useful for various applications.