11. April 2025
Decoding The Enigma Of Large Language Models Unveils Groundbreaking Breakthroughs In Ai Technology
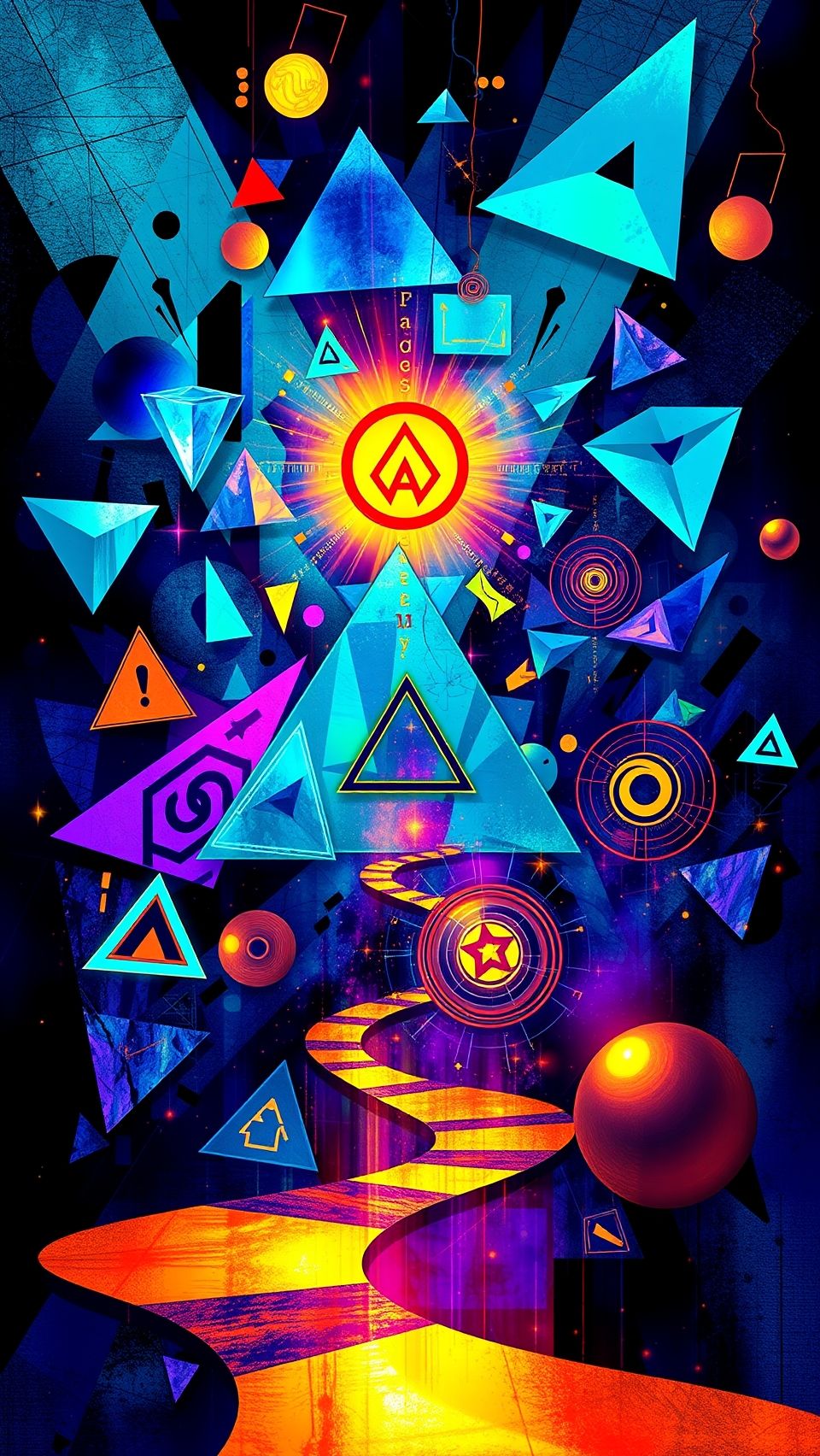
Understanding the Black Box of Large Language Models: Unveiling the Secrets of OLMoTrace
The advent of large language models (LLMs) has revolutionized the way we interact with technology, enabling us to generate human-like responses, summarize complex texts, and even create new content. However, as these models have become increasingly sophisticated, a pressing concern has emerged: understanding precisely how they produce their output.
One major obstacle to enterprise AI adoption is the lack of transparency in how LLMs make decisions. Without a clear understanding of how these models work, it’s challenging for organizations to trust and deploy them effectively. To address this challenge, the Allen Institute for AI (Ai2) has launched an open-source effort called OLMoTrace, which aims to provide a direct window into the relationship between model outputs and training data.
OLMo is an acronym for Open Language Model, and Ai2’s family of open-source LLMs. The recently released OLMo 2 32B model is available on the Ai2 Playground site, allowing users to try out OLMoTrace with ease. The open-source code is also freely available on GitHub, making it accessible to anyone who wants to contribute or experiment with the technology.
OLMoTrace offers a more direct and insightful approach than existing approaches like confidence scores or retrieval-augmented generation (RAG). By analyzing long, unique text sequences in model outputs and matching them with specific documents from the training corpus, OLMoTrace identifies where and how the model learned the information it’s using. This technology provides tangible evidence of AI decision-making, enabling users to make their own informed judgments about model outputs.
“Models can be overconfident of the stuff they generate,” notes Jiacheng Liu, researcher at Ai2. “The confidence scores are usually inflated, which is what academics call a calibration error. Instead of relying on another potentially misleading score, OLMoTrace provides direct evidence of the model’s learning source.” This approach makes OLMoTrace more immediately useful for enterprise applications, as it doesn’t require deep expertise in neural network architecture to interpret the results.
OLMoTrace offers several critical capabilities for enterprise AI teams:
- Fact-checking model outputs against original sources: This feature allows users to verify the accuracy of model-generated content by comparing it with its training data.
- Understanding the origins of hallucinations: By analyzing OLMoTrace output, users can identify where and how models may have generated incorrect or misleading information.
- Improving model debugging: OLMoTrace helps organizations identify problematic patterns in their models, making it easier to debug and improve them.
- Enhancing regulatory compliance: The technology enables data traceability, which is critical for regulated industries where algorithmic transparency is increasingly mandated.
The Ai2 team has already used OLMoTrace to identify and correct issues with their own models. “We are already using it to improve our training data,” Liu reveals. “When we built OLMo 2 and started our training, through OLMoTrace, we found out that some of the post-training data was not good.”
As AI governance frameworks continue to evolve globally, tools like OLMoTrace will likely become essential components of enterprise AI stacks, particularly in regulated industries where algorithmic transparency is increasingly mandated. By providing a practical path to implementing more trustworthy and explainable AI systems, OLMoTrace offers a promising solution for organizations seeking to harness the power of large language models.
In conclusion, OLMoTrace represents a significant step toward more accountable enterprise AI systems. Its ability to provide direct insight into model outputs and training data has far-reaching implications for industries that rely on AI-driven decision-making.