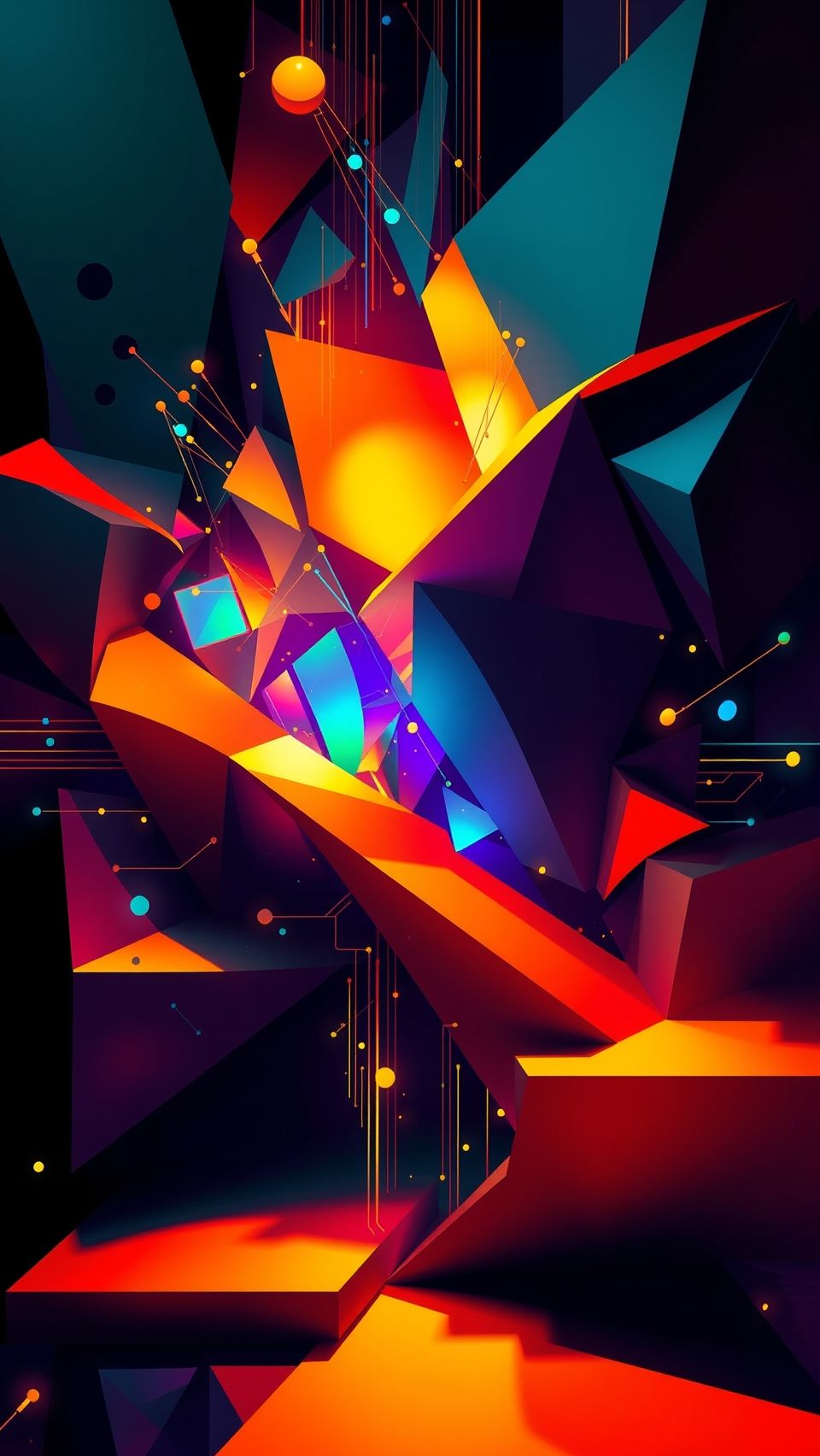
Unlocking the Potential of AI: The Critical Role of Data Quality Management
Data quality management (DQM) is a critical aspect of leveraging artificial intelligence (AI) in today’s fast-evolving business landscape. By implementing DQM practices and utilizing advanced integration tools, organizations can ensure that their data meets the required standards for accuracy and precision, ultimately driving informed decision-making.
A well-executed DQM process provides a “context-specific process for improving the fitness of data used for analysis and decision-making,” according to SAS Institute. This approach enables organizations to identify and address quality issues early on, preventing potential pitfalls and maximizing the effectiveness of their AI models.
Companies are beginning to realize that properly managed data is an asset of potentially limitless potential. A recent study by Harvard Review highlights the growing importance of data in the AI ecosystem: “Data unlocks that potential.”
Effective DQM entails several key areas, including validity, accuracy and precision, redundancy erasure, consistency, and timeliness. By addressing these areas, organizations can enhance their data quality and support the development of more effective AI models.
To achieve this level of DQM, businesses can leverage several key practices, such as data profiling, data cleansing, data standardization, data quality assessment, and data enrichment. Data integration is also critical, as combining data from various sources across the organization creates a unified and comprehensive dataset that supports the development of more effective AI models.
Leading businesses overcome challenges in data integration by leveraging advanced data integration tools, such as IBM’s DataStage, Kore Integrate, and Oracle Data Integrator, which offer comprehensive DQM features. These tools help businesses profile, cleanse, standardize, enrich, and assess data throughout the integration process, creating a single source of accessible and reliable data for AI models to use.
Ultimately, high-quality data leads to high-performing AI – unlocking the full potential of this powerful technology. By implementing DQM practices and utilizing integration tools, businesses can improve both their data quality and AI outcomes.